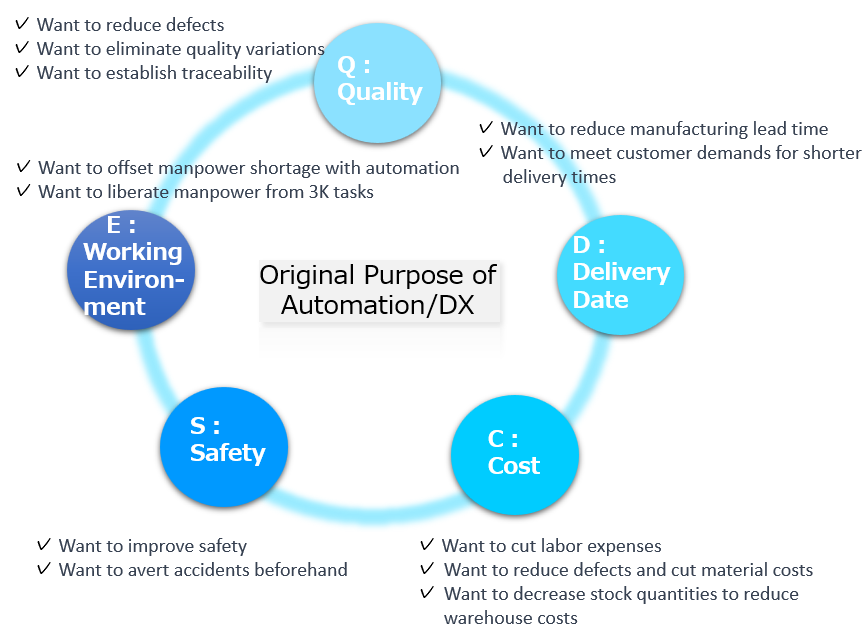
Enhancing competitiveness and establishing an efficient production system in manufacturing industries are achieved through the incorporation of evolving technologies.
Among these, the automation of product identification through the utilization of image processing technology stands as a significant means to achieve quality improvement and cost reduction.
On the other hand, it's crucial to note that "automation is a means, not an end."
When considering the automation of identification using image processing technology, the first step is to clarify the objectives to be achieved through its implementation.
If technology is implemented without a clear purpose, there is a high risk that the intended effects will not be achieved, leading to suboptimal outcomes and potentially resulting in the technology being abandoned, making it a disappointing investment.
Therefore, it is essential to consider the following steps:
Step 1: Clarification of Objectives
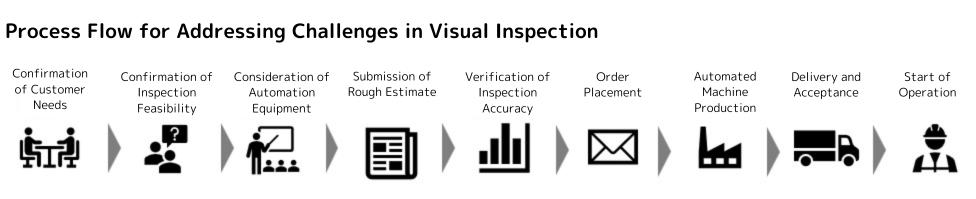
Through automation, it is essential to first clarify what you want to achieve and then determine "how far to automate."
For instance, if the goal is to improve quality by reducing variability in inspections conducted by humans, it may be more cost-effective to have humans handle product input, transportation, and sorting rather than investing heavily in equipment for these tasks.
Conversely, if obtaining inspection personnel is difficult and unmanned inspection is desired, then equipment investment for tasks including product input, transportation, and sorting becomes necessary.
Step 2: Confirmation of Inspection Feasibility for Target Products
Accurately performing determinations using image processing requires checking for any constraints on the positional relationship between the camera/lighting equipment / product. For instance, when photographing to detect minute defects in a product, it is crucial to consider which part of the product to capture, which camera and lighting to use, and how these should be positioned and at what distance.
Step 3: Confirming Operations in Preceding and Subsequent Processes and During Determination Operations
Considering the actual implementation of the system in the production site, factors such as cycle time in preceding and subsequent processes, frequency of product changeovers, range of operations entrusted to operators, handling of defective products identified, etc., are taken into account to compile constraints to consider when evaluating automated systems.
Additionally, the following points are also considered.
Integration with existing production lines: How the automation system will be integrated with existing production facilities and processes.
Data management and analysis: Methods for managing collected image data and mechanisms for data analysis after defective product detection.
Scalability and future-proofing: Whether the system can adapt flexibly if the production line changes or expands in the future.
Step 4: Requirement Definition
Based on the constraints and requirements identified in Steps 2 and 3, the designer will develop a design proposal for image processing determination based on this requirement definition.
Step 5: Implementation of Design
The constraints and requests identified in Steps 2 and 3 are compiled into a requirements definition, and designers then create a design proposal for the image processing determination system based on this requirements definition.
Basic Design: This involves clarifying how the requirements defined in the requirements definition can be systematically realized and designing a conceptual configuration.
In many cases, after completing the basic design, obtaining a rough estimate is essential before transitioning to the detailed design phase, which typically incurs significant effort.
The company considering implementation evaluates whether the investment is justified based on this quotation and the preliminary design proposal.
Detailed Design: Based on the content of the basic design, this phase involves creating structural diagrams of the system, determining the components to be used, and considering control and operational specifications.
Summary
This is applicable not only to image processing determination systems but also to other implementations.
In many cases, what is considered "obvious" within a company "may not be obvious" externally. The extraction of constraints related to image processing is essential, but equally crucial is the articulation of the company's "tacit knowledge" when considering the entire process.
Ensuring this knowledge is translated into constraints and requirements is crucial to avoid a post-implementation realization of "this isn't what we expected."